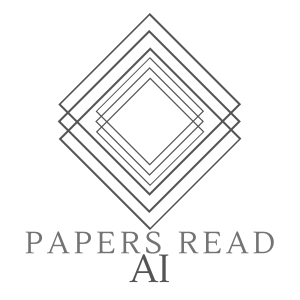
Introduced by Hinton et al. in 2012, dropout has stood the test of time as a regularizer for preventing overfitting in neural networks. In this study, we demonstrate that dropout can also mitigate underfitting when used at the start of training. During the early phase, we find dropout reduces the directional variance of gradients across mini-batches and helps align the mini-batch gradients with the entire dataset's gradient. This helps counteract the stochasticity of SGD and limit the influence of individual batches on model training. Our findings lead us to a solution for improving performance in underfitting models. 2023: Zhuang Liu, Zhi Xu, Joseph Jin, Zhiqiang Shen, Trevor Darrell https://arxiv.org/pdf/2303.01500v1.pdf
Version: 20240320
Comments (0)
To leave or reply to comments, please download free Podbean or
No Comments
To leave or reply to comments,
please download free Podbean App.