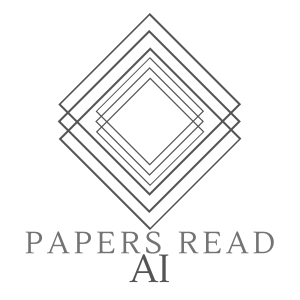
We argue that representations in AI models, particularly deep networks, are converging. First, we survey many examples of convergence in the literature: over time and across multiple domains, the ways by which different neural networks represent data are becoming more aligned. Next, we demonstrate convergence across data modalities: as vision models and language models get larger, they measure distance between datapoints in a more and more alike way. We hypothesize that this convergence is driving toward a shared statistical model of reality, akin to Plato's concept of an ideal reality. We term such a representation the platonic representation and discuss several possible selective pressures toward it. Finally, we discuss the implications of these trends, their limitations, and counterexamples to our analysis.
2024: Minyoung Huh, Brian Cheung, Tongzhou Wang, Phillip Isola
https://arxiv.org/pdf/2405.07987
Comments (0)
To leave or reply to comments, please download free Podbean or
No Comments
To leave or reply to comments,
please download free Podbean App.