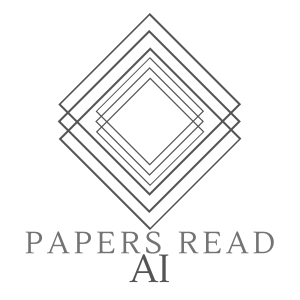
Recent studies show that Vision Transformers (ViTs) exhibit strong robustness against various corruptions. Although this property is partly attributed to the self-attention mechanism, there is still a lack of systematic understanding. In this paper, we examine the role of self-attention in learning robust representations. Our study is motivated by the intriguing properties of the emerging visual grouping in Vision Transformers, which indicates that self-attention may promote robustness through improved mid-level representations. We further propose a family of fully attentional networks (FANs) that strengthen this capability by incorporating an attentional channel processing design. We validate the design com-prehensively on various hierarchical backbones. 2022: Daquan Zhou, Zhiding Yu, Enze Xie, Chaowei Xiao, Anima Anandkumar, Jiashi Feng, J. Álvarez Ranked #2 on Domain Generalization on ImageNet-C (using extra training data) https://arxiv.org/pdf/2204.12451v2.pdf
No comments yet. Be the first to say something!