Wednesday May 31, 2023
CodeT5+: Open Code Large Language Models for Code Understanding and Generation
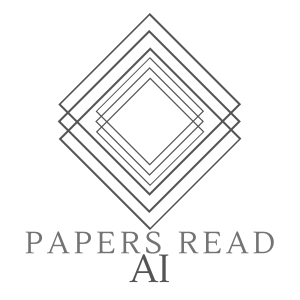
Large language models (LLMs) pretrained on vast source code have achieved prominent progress in code intelligence. However, existing code LLMs have two main limitations in terms of architecture and pretraining tasks. First, they often adopt a specific architecture (encoder-only or decoder-only) or rely on a unified encoder-decoder network for different downstream tasks. The former paradigm is limited by inflexibility in applications while in the latter, the model is treated as a single system for all tasks, leading to suboptimal performance on a subset of tasks. Secondly, they often employ a limited set of pretraining objectives which might not be relevant to some downstream tasks and hence result in substantial performance degrade. To address these limitations, we propose ``CodeT5+'', a family of encoder-decoder LLMs for code in which component modules can be flexibly combined to suit a wide range of downstream code tasks. 2023: Yue Wang, Hung Le, Akhilesh Deepak Gotmare, Nghi D. Q. Bui, Junnan Li, Steven Hoi Ranked #1 on Code Search on CodeXGLUE - AdvTest https://arxiv.org/pdf/2305.07922v1.pdf
Comments (0)
To leave or reply to comments, please download free Podbean or
No Comments
To leave or reply to comments,
please download free Podbean App.