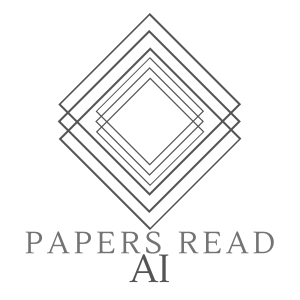
This paper studies a simple extension of image-based Masked Autoencoders (MAE) [1] to self-supervised representation learning from audio spectrograms. Following the Transformer encoder-decoder design in MAE, our Audio-MAE first encodes audio spectrogram patches with a high masking ratio, feeding only the non-masked tokens through encoder layers. The decoder then re-orders and decodes the encoded context padded with mask tokens, in order to reconstruct the input spectrogram. We find it beneficial to incorporate local window attention in the decoder, as audio spectrograms are highly correlated in local time and frequency bands. 2022: Po-Yao Huang, Hu Xu, Juncheng Billy Li, Alexei Baevski, Michael Auli, Wojciech Galuba, Florian Metze, Christoph Feichtenhofer Ranked #6 on Audio Classification on AudioSet https://arxiv.org/pdf/2207.06405v2.pdf
Comments (0)
To leave or reply to comments, please download free Podbean or
No Comments
To leave or reply to comments,
please download free Podbean App.