Monday Mar 27, 2023
SmoothQuant: Accurate and Efficient Post-Training Quantization for Large Language Models
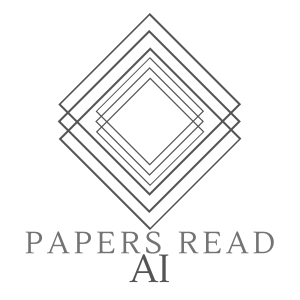
Large language models (LLMs) show excellent performance but are compute- and memory-intensive. Quantization can reduce memory and accelerate inference. However, for LLMs beyond 100 billion parameters, existing methods cannot maintain accuracy or do not run efficiently on hardware. We propose SmoothQuant, a training-free, accuracy-preserving, and general-purpose post-training quantization (PTQ) solution to enable 8-bit weight, 8-bit activation (W8A8) quantization for LLMs. Based on the fact that weights are easy to quantize while activations are not, SmoothQuant smooths the activation outliers by offline migrating the quantization difficulty from activations to weights with a mathematically equivalent transformation. 2022: Guangxuan Xiao, Ji Lin, Mickael Seznec, Julien Demouth, Song Han https://arxiv.org/pdf/2211.10438v4.pdf
Comments (0)
To leave or reply to comments, please download free Podbean or
No Comments
To leave or reply to comments,
please download free Podbean App.