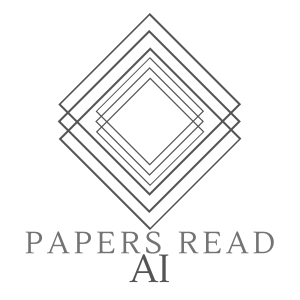
Designed to learn long-range interactions on sequential data, transformers continue to show state-of-the-art results on a wide variety of tasks. In contrast to CNNs, they contain no inductive bias that prioritizes local interactions. This makes them expressive, but also computationally infeasible for long sequences, such as high-resolution images. We demonstrate how combining the effectiveness of the inductive bias of CNNs with the expressivity of transformers enables them to model and thereby synthesize high-resolution images. We show how to (i) use CNNs to learn a context-rich vocabulary of image constituents, and in turn (ii) utilize transformers to efficiently model their composition within high-resolution images. 2020: Patrick Esser, Robin Rombach, B. Ommer Ranked #3 on Text-to-Image Generation on LHQC https://arxiv.org/pdf/2012.09841v3.pdf
No comments yet. Be the first to say something!