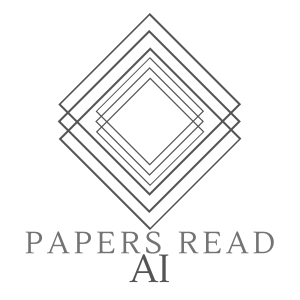
Convolutional models have been widely used in multiple domains. However, most existing models only use local convolution , making the model unable to handle long-range dependency efficiently. Attention overcomes this problem by aggregating global information based on the pair-wise attention score but also makes the computational complexity quadratic to the sequence length. S4 can be efficiently implemented as a global convolutional model whose kernel size equals the input sequence length. With Fast Fourier Transform, S4 can model much longer sequences than Transformers and achieve significant gains over SoTA on several long-range tasks. Despite its empirical success, S4 is involved. It requires sophis-ticated parameterization and initialization schemes that combine the wisdom from several prior works. As a result, S4 is less intuitive and hard to use for researchers with limited prior knowledge. Here we aim to demystify S4 and extract basic principles that contribute to the success of S4 as a global convolutional model.
2022: Yuhong Li, Tianle Cai, Yi Zhang, De-huai Chen, Debadeepta Dey https://arxiv.org/pdf/2210.09298v1.pdf
No comments yet. Be the first to say something!